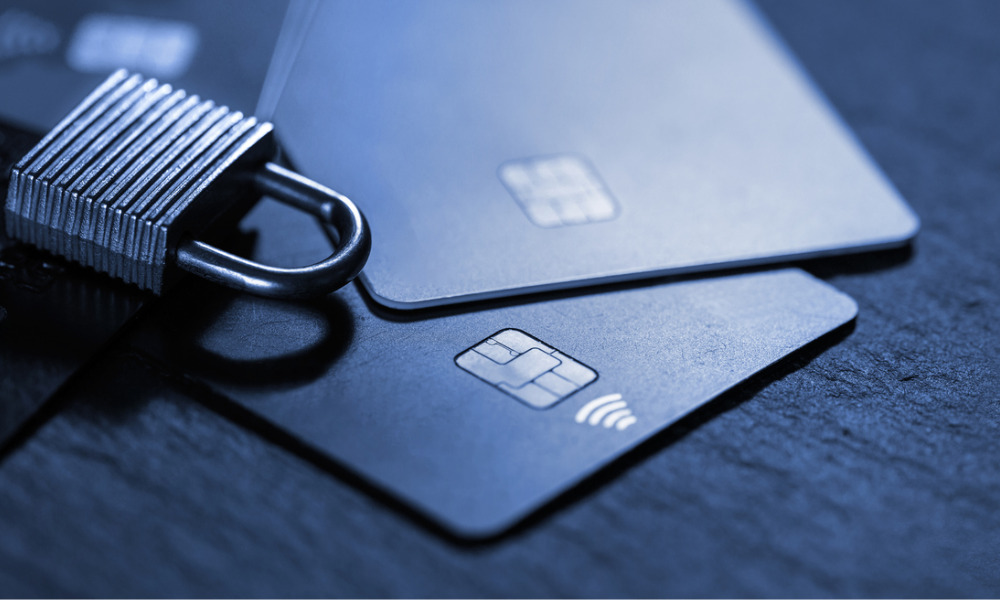
The financial industry has long been an attractive target for fraudsters. Payment fraud, in particular, has seen a dramatic rise with the growth of e-commerce, digital wallets, and online banking. Traditional methods of fraud detection, like rule-based systems and manual monitoring, have struggled to keep up with the ever-evolving tactics of criminals. However, with the rapid advancement of artificial intelligence (AI), the landscape of payment fraud detection is undergoing a major transformation. AI technologies, such as machine learning and deep learning, are proving to be game-changers in the fight against payment fraud.
In this blog, we will explore the profound impact AI is having on payment fraud detection, how it is reshaping fraud prevention strategies, and why businesses and financial institutions need to embrace AI to stay ahead of fraudulent activities. If you’re interested in how these innovations can bolster your fraud prevention mechanisms, click here to explore our AI-powered fraud detection solutions.
The Rise of Payment Fraud and the Need for Innovation
As digital payment systems become more sophisticated, so do the methods used by fraudsters. The shift from physical to digital transactions has made it easier for criminals to carry out fraudulent activities without being physically present. Cybercriminals use various tactics, including identity theft, account takeovers, and phishing attacks, to exploit vulnerabilities in payment systems. The rise of online and mobile payments, along with the increase in cross-border transactions, has opened up new avenues for fraudsters, making traditional fraud detection methods insufficient.
This has led to a pressing need for more efficient and effective ways to detect and prevent payment fraud. Traditional methods such as static rules-based systems, which rely on predefined patterns and thresholds to identify fraud, have shown limitations. They struggle to detect new, evolving fraud techniques, and often generate high volumes of false positives. Financial institutions and businesses are therefore turning to AI to address these challenges and improve fraud detection systems.
How AI is Transforming Payment Fraud Detection
Artificial intelligence, particularly machine learning (ML), is revolutionizing payment fraud detection by introducing more adaptive, predictive, and dynamic fraud detection models. AI systems can learn from historical transaction data, identify patterns, and detect anomalies that may signify fraudulent activity. Unlike traditional systems, AI does not require predefined rules, which allows it to continuously adapt to new types of fraud. Here are some of the key ways AI is making a difference in the world of payment fraud detection:
-
Machine Learning for Real-Time Fraud Detection
Machine learning algorithms are one of the most powerful tools AI has brought to fraud detection. These algorithms can analyze vast amounts of transaction data in real-time and identify unusual patterns that may indicate fraudulent behavior. Unlike rule-based systems, machine learning algorithms can evolve and improve over time, becoming more accurate as they are exposed to new data.
For example, machine learning models can detect patterns such as sudden changes in spending behavior, mismatched IP addresses, or unusual geographical locations that may indicate stolen account details or card fraud. These systems can also recognize emerging fraud trends by continuously learning from historical transaction data, enabling them to detect fraud as it happens, without relying on predefined rules.
-
Behavioral Biometrics for Fraud Prevention
Another area where AI is making an impact is in the use of behavioral biometrics. Behavioral biometrics focuses on analyzing how users interact with their devices to build unique profiles that can help detect fraudulent activity. For instance, AI can track how a person types, moves the mouse, or swipes on a touchscreen and create a unique “behavioral fingerprint.” If someone else attempts to use the account, the system can compare their behavior to the established profile and flag any discrepancies.
Behavioral biometrics offers a significant advantage because it is difficult for fraudsters to replicate a user’s natural patterns of behavior, even if they have stolen login credentials. By using AI to track these behavioral cues, financial institutions can add an extra layer of protection against identity theft and account takeovers.
-
Predictive Analytics for Fraud Prevention
AI-powered predictive analytics can anticipate fraudulent behavior before it happens. By analyzing historical data, AI models can identify subtle patterns and trends that indicate the likelihood of future fraudulent activities. For example, AI can predict whether a particular transaction is likely to be fraudulent based on the user’s previous transaction history, device information, and behavioral data.
These predictive models can also help financial institutions prioritize which transactions to investigate first. Instead of flagging every transaction that doesn’t match a predefined rule, AI can use predictive analytics to identify high-risk transactions, reducing the number of false positives and improving the efficiency of fraud detection systems.
-
Fraud Pattern Recognition and Anomaly Detection
Fraud detection is largely about spotting anomalies—transactions that deviate from a user’s typical behavior or the expected behavior for a given type of transaction. AI excels in identifying these anomalies by analyzing massive amounts of transactional data and looking for outliers that could indicate fraud.
For example, AI can detect changes in a user’s spending habits, such as an unusually large purchase or transactions in a new country, and flag them for review. It can also identify correlations between seemingly unrelated events, such as a change in location and a sudden increase in transaction volume, which might not be noticeable using traditional detection methods.
In addition, machine learning models can help detect sophisticated fraud schemes by identifying subtle fraud patterns that traditional systems would miss. This is particularly important for combating synthetic fraud, where criminals create fake identities and use them for fraudulent transactions.
-
Natural Language Processing (NLP) for Fraud Detection
Natural Language Processing (NLP) is a subfield of AI that focuses on the interaction between computers and human language. In the context of fraud detection, NLP can be used to analyze text-based data, such as emails, customer service conversations, and transaction descriptions, to detect signs of fraudulent activity.
For example, NLP algorithms can scan emails or customer messages for signs of phishing attacks or other types of social engineering scams. They can also analyze the tone and content of conversations to detect potential fraud attempts, helping financial institutions identify fraud risks before they escalate.
Benefits of AI in Payment Fraud Detection
The integration of AI into payment fraud detection systems offers numerous advantages for businesses and financial institutions, including:
- Improved Accuracy: AI algorithms are capable of learning from vast amounts of transaction data, allowing them to detect fraud more accurately than rule-based systems. This leads to fewer false positives and a reduced likelihood of legitimate transactions being flagged as fraud.
- Faster Response Times: AI systems can detect fraudulent transactions in real-time, allowing businesses and financial institutions to respond quickly and prevent further damage. This is critical for reducing financial losses and protecting customers.
- Adaptability: AI systems continuously improve over time, adapting to new fraud techniques and emerging trends. This adaptability ensures that AI-powered fraud detection systems remain effective, even as fraudsters evolve their tactics.
- Scalability: AI can process and analyze large volumes of transaction data much faster and more efficiently than human analysts, enabling businesses to scale their fraud detection efforts as they grow.
- Cost-Effectiveness: While implementing AI solutions requires upfront investment, the long-term savings in fraud detection and prevention can be significant. By reducing fraud and minimizing the costs of manual monitoring, businesses can achieve a positive return on investment (ROI).
Challenges of AI in Payment Fraud Detection
While AI offers substantial benefits, there are also challenges to consider:
- Data Privacy Concerns: AI systems require vast amounts of data to learn and improve. Ensuring that this data is handled securely and in compliance with privacy regulations, such as the GDPR, is a key concern for many businesses.
- Bias and Fairness: Like all AI systems, fraud detection algorithms can inherit biases from the data they are trained on. This can result in unfair outcomes, such as disproportionately flagging transactions from certain regions or customer demographics as fraudulent. It’s crucial to ensure that AI models are designed and tested to minimize biases.
- Integration Complexity: Integrating AI-driven fraud detection systems with existing payment infrastructures can be complex and costly. Businesses need to invest in the right tools and expertise to ensure a smooth transition and effective implementation.
The Future of AI in Payment Fraud Detection
The role of AI in payment fraud detection will continue to grow, with more sophisticated techniques emerging to stay one step ahead of fraudsters. As AI continues to evolve, we can expect improvements in areas such as biometric authentication, voice recognition, and cross-platform fraud detection.
In the future, AI could even be integrated with blockchain technology to create more secure and transparent payment systems. Blockchain’s decentralized nature and AI’s predictive capabilities could combine to provide real-time fraud detection with unmatched accuracy.
Conclusion
AI is revolutionizing payment fraud detection by offering businesses and financial institutions the ability to detect fraud in real-time, adapt to emerging threats, and reduce false positives. By utilizing machine learning, behavioral biometrics, predictive analytics, and other AI technologies, organizations can stay ahead of fraudsters and protect both their assets and their customers.
As AI continues to evolve, it will become even more integral to combating payment fraud, providing smarter, more efficient solutions for fraud prevention. For businesses looking to stay competitive and secure in the digital age, embracing AI-driven fraud detection is no longer optional—it’s a necessity.